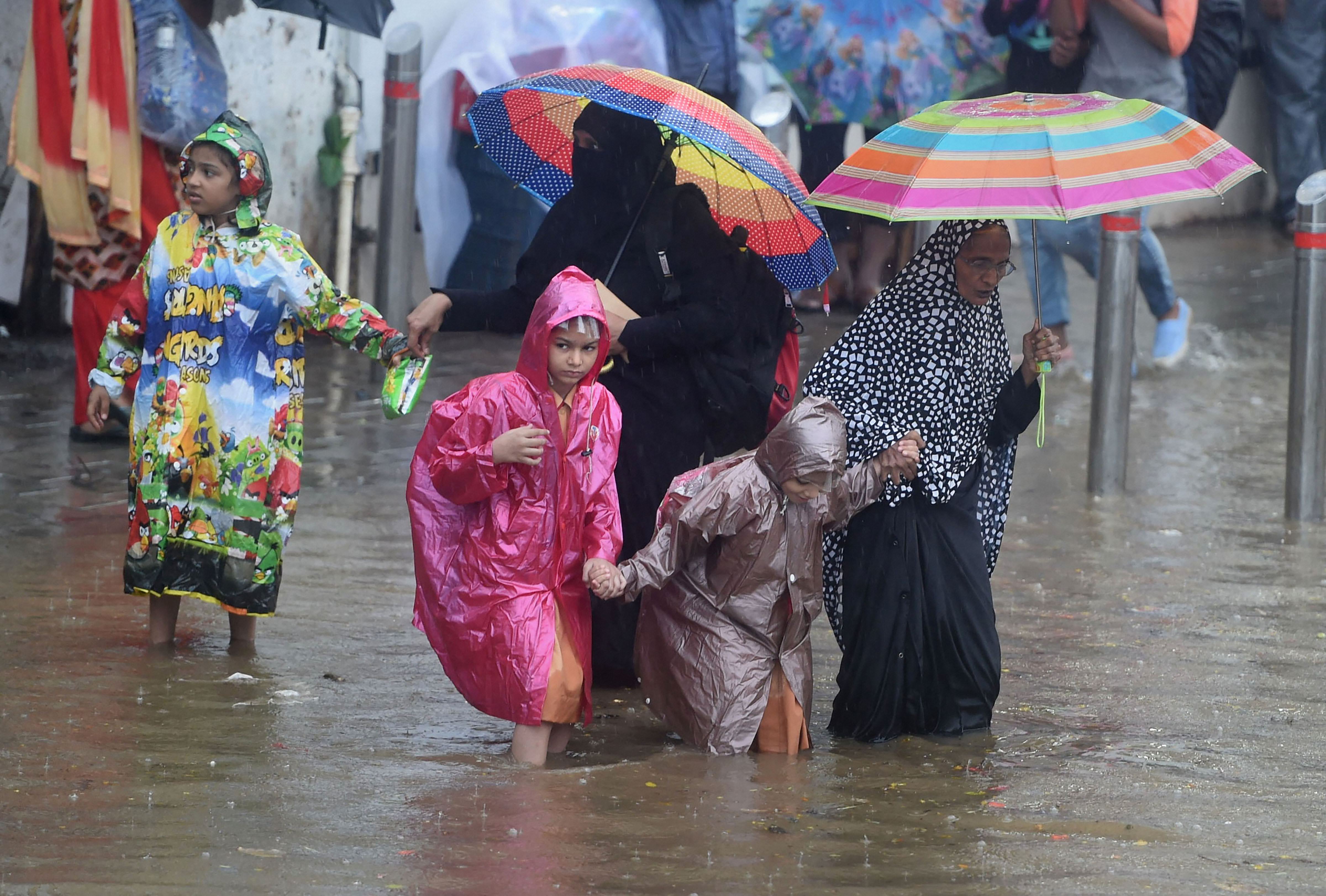
Here is how forecast of extreme rainfall events can be improved
On July 2, the Maharashtra government decided to close private and public schools in Mumbai based on IMD’s rainfall forecast which predicted “intermittent rain in city and suburbs, with heavy to very heavy rainfall at a few places for the next 24 hours.” The 24-hour period from 8:30 am of July 1 to July 2 had seen a total of 375 mm of rain and it was obviously ominous to expect that more heavy rain events would follow.
The 24-hour periods during July 2-3 and July 3-4 received only 34 mm and 4 mm of rain, respectively. IMD was late by a day in calling for an extreme event. Are IMD forecasts of extreme rains later or earlier than actual events on some occasions? This may be the case as indicated by a 2017 study led by a graduate student at IIT-Bombay, Hiteshri Shastri, who has since completed her PhD. The team has developed a method for statistical processing of extreme event forecasts to reduce this shift in time.
Greater precision in forecast of extreme events is critical. The Brihanmumbai Municipal Corporation (BMC) was caught off guard in the deluge of 2005 despite an IMD forecast of heavy rains several days ahead. A post-mortem of that event led to much improved response in 2017 and rapid draining of flooded areas in 2019 is also commendable. While BMC is learning to respond appropriately to forecasts but forecasts themselves need further improvements.
How rainfall is predicted
The Numerical Weather Prediction model (NWP), a computer model that solves complicated equations which determine the evolution of weather for several days is used for forecasting. The IMD employs a numerical model called the Global Ensemble Forecasting System (GEFS) and it has resulted in remarkable improvement in predictions. The prediction of extreme rainfall events, however, remains challenging as by their very nature such events are rare. Work in this area is important, given that India is already experiencing increased heavy and very heavy rainfall events as well a threefold increase in extreme rainfall events.
GEFS does global forecasts at 70 Km resolution – meaning the earth is divided into cubes of 70 Km in the horizontal with 70 layers in the vertical going from the surface to the stratosphere. The capacity of the global version of GEFS for predicting extreme events in India is rather meagre. IMD has, therefore, developed a regional version of the GEFS covering South Asia at a resolution 12 km to downscale global forecasts and is further downscaling on a short period of a day or two to a few hours at 4 km and 1.5 km.
While the skill of rainfall forecasts at short range (day one to three) and medium range (day three to 10) have shown some improvements, the probability of detecting extreme events – say rainfall in the 99th or 95th percentile of the expected rain at a given location for a given day – is still very poor. The ‘false alarm ratio’ – predicting extreme events when none occur – is also high. What the IITB study discovered is that the model tends to produce extreme rains one to two day before or one to two days after the event occurs in reality. The July 3 warning following the July 2 heavy rains is a clear example of such shifts in time between forecasts and actual events in the current forecast system.
Overcoming challenges in forecasting local rains
The IITB study exploits the relation between large-scale circulation features associated with such heavy rain events to statistically process GEFS forecasts to increase the ‘probability of detection’ and reduce the ‘false alarm ratio’. Large-scale features are easier to forecast than local heavy rain events which can be caused by depressions originating in the Arabian Sea or the Bay of Bengal, or with local heating of land, or by westerly disturbances in some parts of the country like the northwest.
Increasing model resolution may offer further improvements but running such high resolution models is nearly impossible for rapid forecasting of local heavy rain events. Using empirical relations between large-scale features and extreme rain events, statistical forecasts of which quantile the rainfall event may fall into can be performed quickly for up to day 5.
Combining model forecasts of large-scale features with known past values of temperature, humidity and winds at different pressure levels from the surface to the upper atmosphere, the statistical model can add demonstrable skill to predictions of heavy rain events. These methods are popularly known as ‘quantitative precipitation forecasting’ or QPF and they are computationally very economical compared to running very high-resolution models for particular regions or cities.
Tuning into QPF for better forecast
Statistical QPF models are trained and validated for a past period based on past forecasts and observations, and then applied to actual forecasting in real time. Forecast experiments by the IITB team for 2006 and 2007 showed that their QPF increased the ‘probability of detection’ using GEFS from 20 per cent to 90 per cent and when a five-day period was considered, it reduced the ‘false alarm ratio’ by 90 per cent. While GEFS detected only six of 29 events during this period, the QPF could identify 17 of 29 events.
Empirical relations between large-scale circulation and local heavy rains can change because of global warming or other factors such as land use. But QPF could still be very be valuable for a country like India which needs to use all the tools available. Besides, the changing relations can be dealt with by training and validating the QPF model each year prior to the monsoon season and keeping it at the current state of statistical relations between the large-scale patterns and extreme events. By adding QPF to IMD forecasts, there can be more reliable extreme event forecasts which can help agencies like BMC making life-saving decisions.
(The writer is a professor of atmospheric and oceanic science and earth system science at the University of Maryland. He is currently a visiting professor at IIT Bombay)
(The article was first published in India Science Wire)