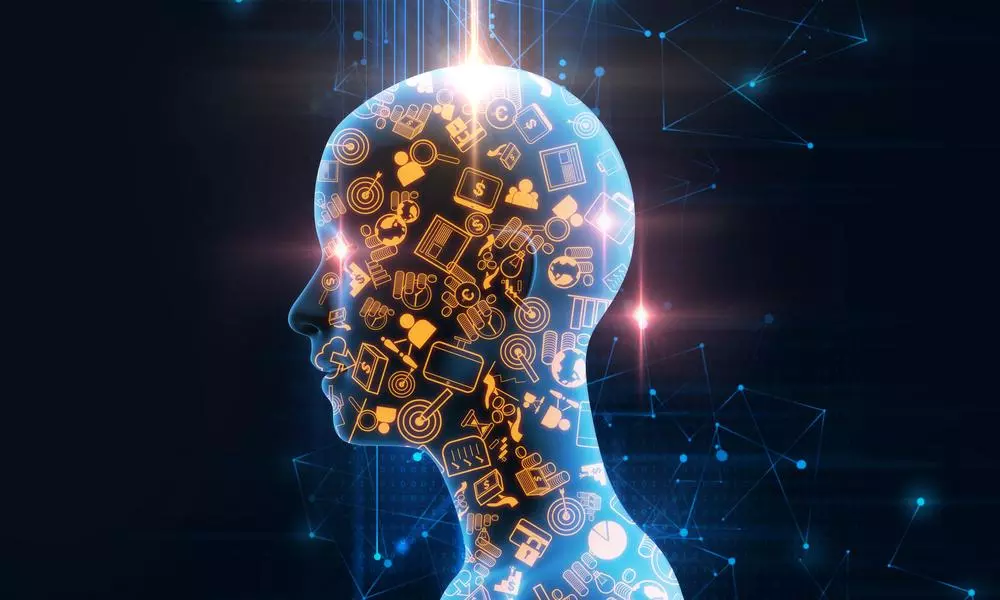
Why ChatGPT isn’t conscious – but future AI systems might be
No current system comes anywhere near the bar for consciousness – but at the same time, there’s no obvious reason future systems won’t become truly aware
In June 2022, Google engineer Blake Lemoine made headlines by claiming the company’s LaMDA chatbot had achieved sentience. The software had the conversational ability of a precocious seven-year-old, Lemoine said, and we should assume it possessed a similar awareness of the world.
LaMDA, later released to the public as Bard, is powered by a “large language model” (LLM) of the kind that also forms the engine of OpenAI’s ChatGPT bot. Other big tech companies are rushing to deploy similar technology.
Hundreds of millions of people have now had the chance to play with LLMs, but few seem to believe they are conscious. Instead, in linguist and data scientist Emily Bender’s poetic phrase, they are “stochastic parrots”, which chatter convincingly without understanding. But what about the next generation of artificial intelligence (AI) systems, and the one after that?
Our team of philosophers, neuroscientists and computer scientists looked to current scientific theories of how human consciousness works to draw up a list of basic computational properties that any hypothetically conscious system would likely need to possess. In our view, no current system comes anywhere near the bar for consciousness – but at the same time, there’s no obvious reason future systems won’t become truly aware.
Finding indicators
Since computing pioneer Alan Turing proposed his “Imitation Game” in 1950, the ability to successfully impersonate a human in conversation has often been taken as a reliable marker of consciousness. This is usually because the task has seemed so difficult it must require consciousness.
However, as with chess computer Deep Blue’s 1997 defeat of grandmaster Gary Kasparov, the conversational fluency of LLMs may just move the goalposts. Is there a principled way to approach the question of AI consciousness that does not rely on our intuitions about what is difficult or special about human cognition?
Our recent white paper aims to do just that. We compared current scientific theories of what makes humans conscious to compile a list of “indicator properties” that could then be applied to AI systems.
We don’t think systems that possess the indicator properties are definitely conscious, but the more indicators, the more seriously we should take claims of AI consciousness.
The computational processes behind consciousness
What sort of indicators were we looking for? We avoided overt behavioural criteria – such as being able to hold conversations with people – because these tend to be both human-centric and easy to fake.
Instead, we looked at theories of the computational processes that support consciousness in the human brain. These can tell us about the sort of information-processing needed to support subjective experience.
“Global workspace theories”, for example, postulate that consciousness arises from the presence of a capacity-limited bottleneck which collates information from all parts of the brain and selects information to make globally available. “Recurrent processing theories” emphasise the role of feedback from later processes to earlier ones.
Each theory in turn suggests more specific indicators. Our final list contains 14 indicators, each focusing on an aspect of how systems work rather than how they behave.
Indicators of consciousness
Different theories of consciousness suggest some basic properties we might expect a conscious system to have.
Recurrent processing theory
Input modules using algorithmic recurrence
Input modules generating organised, integrated perceptual representations
Global workspace theory
Multiple specialised systems capable of operating in parallel (modules)
Limited capacity workspace, entailing a bottleneck in information flow and a selective attention mechanism
Global broadcast: availability of information in the workspace to all modules
State-dependent attention, giving rise to the capacity to use the workspace to query modules in succession to perform complex tasks
Computational higher-order theories
Generative, top-down or noisy perception modules
Metacognitive monitoring distinguishing reliable perceptual representations from noise
Agency guided by a general belief-formation and action selection system, and a strong disposition to update beliefs in accordance with the outputs of metacognitive monitoring
Sparse and smooth coding generating a “quality space”
Attention schema theory
A predictive model representing and enabling control over the current state of attention
Predictive processing
Input modules using predictive coding
Agency and embodiment
Agency: learning from feedback and selecting outputs so as to pursue goals, especially where this involves flexible responsiveness to competing goals
Embodiment: modelling output-input contingencies, including some systematic effects, and using this model in perception or control
No reason to think current systems are conscious
How do current technologies stack up? Our analysis suggests there is no reason to think current AI systems are conscious.
Some do meet a few of the indicators. Systems using the transformer architecture, a kind of machine-learning model behind ChatGPT and similar tools, meet three of the “global workspace” indicators, but lack the crucial ability for global rebroadcast. They also fail to satisfy most of the other indicators.
So, despite ChatGPT’s impressive conversational abilities, there is probably nobody home inside. Other architectures similarly meet at best a handful of criteria.
Most current architectures only meet a few of the indicators at most. However, for most of the indicators, there is at least one current architecture that meets it.
This suggests there are no obvious, in-principle technical barriers to building AI systems that satisfy most or all of the indicators.
It is probably a matter of when rather than if some such system is built. Of course, plenty of questions will still remain when that happens.
Beyond human consciousness
The scientific theories we canvass (and the authors of the paper!) don’t always agree with one another. We used a list of indicators rather than strict criteria to acknowledge that fact. This can be a powerful methodology in the face of scientific uncertainty.
We were inspired by similar debates about animal consciousness. Most of us think at least some nonhuman animals are conscious, despite the fact they cannot converse with us about what they’re feeling.
A 2021 report from the London School of Economics arguing that cephalopods such as octopuses likely feel pain was instrumental in changing UK animal ethics policy. A focus on structural features has the surprising consequence that even some simple animals, like insects, might even possess a minimal form of consciousness.
Our report does not make recommendations for what to do with conscious AI. This question will become more pressing as AI systems inevitably become more powerful and widely deployed.
Our indicators will not be the last word – but we hope they will become a first step in tackling this tricky question in a scientifically grounded way.